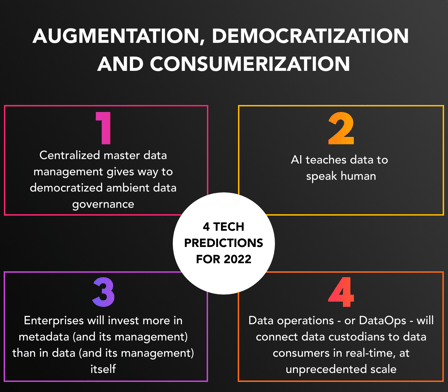
2021 has certainly been an eventful year, with even the most conservative new technology adopter organizations being forced to take a digital leap that certainly would have been unimaginable had it not been for the unprecedented global pandemic acting as catalyst for digital-by-necessity.
Read also: The true digital industry 4.0
In this year’s tech predictions, we are focusing on a very specific field of technology: industrial data management. More specifically still, we are zooming in on how cloud data storage will finally be connected to industrial use case solving at scale, unlocked by advances in what has long been an elusive yet critical “hidden layer” between data storage and application logic. Before we start, two notes to inform readers:
-
For those looking for more general technology predictions, there is no shortage of well-researched examples published around this time of the year by the likes of IDC, Forrester and Gartner as examples; and
-
This is not another vague prediction on how digital twins will transform industry (there is equally no shortage of great research and predictions on this topic dating back a good decade at the convenience of any web search engine)
Augmentation, Democratisation and Consumerization
Searching for good terms to categorise and summarise our 4 industrial data management tech niche predictions for 2022, we surprisingly quickly arrived at augmentation, democratisation and consumerization. Enough prologue. Please find below our predictions for industrial data management tech niche’s strategic technology trends that we at Cognite believe will drive significant disruption and opportunity over the next 3 years — if not even sooner.
![]() |
Corporate data governance gives way to democratized ambient data governance |
Organizations need to be able to apply different styles of governance for different types of data and analytics. Data governance topics such as data quality and data lineage transparency move from being a centralized, use case agnostic practice; to becoming intrinsically linked to actual data consumers and their data-driven use case requirements. Through this paradigm shift, data governance — and master data management — is no longer a roadblock (or tax) on faster use case execution and greater use case development team autonomy, but becomes infused into use case solving itself. As first step, we predict that data discovery will become an indistinguishable part of data governance.
Learn more: The truth about industrial digitalization
What does this mean?
Use case specific data models with scalable data templates become the new focal point of data governance. Traditional enterprise data custodian roles evolve into data-driven product portfolio management roles. Data consumers — such as data scientist, application developers, and SMEs — will take lead in defining and configuring data governance for their use cases themselves.
![]() |
AI teaches ET/OT/IT data to speak human |
AI-driven active metadata creation permeates industrial data management, shifting the emphasis from data storage and cataloging to a true human data discovery experiences. For application developers and data scientist, understanding — and handling — industrial data is not as straight forward as dealing with most tabular data. What is even more difficult (for all who are not SMEs with years of intimate experience with the asset) is understanding the context of, as well as further contextually related, industrial data. Using NLP, OCR, computer vision, trained ontologies and graph data models, ET/OT/IT will be automatically contextualized for intuitive human as well as programmatic discovery and analysis.
Learn more: Data management (r)evolution in the age of AI and the citizen data scientist
What does this mean?
The same shift that has already taken place on the consumer side e.g. e-commerce — wherein the primary navigation has shifted from product catalog navigation to automated content recommendations — will transform enterprise data discovery experiences, enabling new data consumers to self-service access large, varied and complex data sets for the first time, unlocking citizen data scientist and citizen developer innovation potential at scale
![]() |
Enterprises will invest more in metadata (and its management) than in data (and its management) itself |
Data science teams are scrambling to convert the contents of their data warehouses and data lakes into business value (not that DS is the only data consumer class by far, but they are the ones with the highest expectations to delivery transformative solutions.) Data lakes have become data swamps. To fulfill the market’s demand for human understandable data, despite an increasingly complex data landscape, metadata management is stepping up, driven by expansion of Chief Data Office (CDO) mandate to including metadata ownership (from the CIO).
Read also: What is data fabric and how does it complement my data warehouse?
What does this mean?
Whether through manual tagging of images or advanced scalable AI-driven
data set matching to uncover data relationships or OCR/NLP methods to convert unstructured data into structured data, the focus on — and value of — metadata is set to exceed that of the data itself. Automated data contextualization is at the centre of modern metadata curation.
|
Data operations — or DataOps — will connect data managers to data consumers in real-time, at unprecedented scale |
DataOps is collaborative data management for the AI era. The convergence of data management with data analytics continues to accelerate, driven by an exponential growth in data literacy aspiring data consumers. Seamless data operationalization across all workflow steps ranging from data sources to live applications becomes the new ‘data trapped in source system siloes’ challenge for digital and innovation leaders.
Learn more: DataOps: A transformative new approach to data ROI
What does this mean?
Organisations who want to get ahead of the curve are investing in DataOps as the ability to enable solutions, develop data products, and activate data for business value across all technology tier from infrastructure to experience.