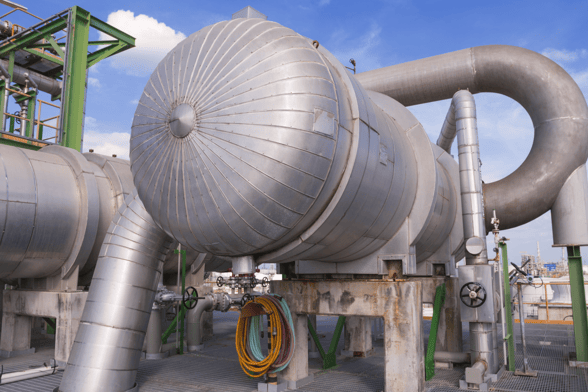
Digital transformation presents a major opportunity for downstream operators to improve key business drivers in their operations. With easy access to data that flows freely across the organization, operators can make informed decisions about where to prioritize maintenance investments, maximizing plant performance and extending equipment life.
Working with major players closely in heavy asset industries, we have seen that the data trapped in complex, siloed systems presents a key challenge to extracting useful insights.
Born from industry, Cognite specializes in liberating the data locked in disparate IT and OT data sources, and contextualizing it for easier creation and deployment of analytical models and business applications.
This article explains how Cognite’s products can help operators and plant owners plan and carry out effective maintenance and inspection activities while gaining much deeper insight into asset integrity. Our customers, including Aker BP and Wintershall Dea, are using Cognite Data Fusion® (CDF) and other products to effectively monitor asset integrity, anticipate unexpected shutdown, and boost plant uptime with a state-of-the-art root-cause analysis system powered by physics-guided machine learning.
Read more: Downstream Digitalization: Boosting field workers’ health, safety, and productivity
Operators lose money and degrade equipment life with outdated maintenance activities
Despite the rise of tools and techniques to enable predictive maintenance, most downstream operators waste time and money on calendar-based maintenance that doesn’t increase the lifetime of assets. Calendar-based maintenance of equipment leads to inefficiencies, as the condition of the equipment can’t be accounted for in real time, occasionally resulting in unexpected and costly reactive maintenance and, more importantly, downtime.
Yet downstream operators already collect massive amounts of data about their equipment, including vibration and temperature measurements for the equipment body, shaft power consumption, and fluid measurements such as flow rate, pressure, and temperature. However, refineries often lack an enablement layer that integrates data from disparate sources and correlates it to failure events. Even if the refinery tries to manually get these insights, the process of integrating and standardizing data from different sources typically involves a considerable amount of time and effort from skilled workers -- time which could have been invested in carrying out the actual analysis.
As an added wrinkle, maintenance teams are often tasked with monitoring and maintaining many machines simultaneously. It can be challenging to aggregate and monitor data across many different machine brands, models, etc. Even with machine data, “alarm fatigue” often overwhelms the teams, leading to reactive decision-making. Furthermore, there are examples of equipment specific to refineries that simply can’t be fully monitored through live and historical data alone. For instance, suboptimal reboiler operations caused by rapid fouling can lead to large performance losses for refineries and require augmented data to facilitate data analytics and predictive maintenance.
Increase equipment efficiency and life with a pre-emptive approach to maintenance
Cognite’s products liberate data from different source systems and create a holistic data model with contextualized data that helps plant operators optimize how they conduct maintenance of their critical equipment. This results in increased throughout, less unplanned downtime, improved maintenance planning, and reduced costs. By moving to a more data-driven approach to maintenance, Cognite’s customers are also able to reduce their energy consumption, as healthy equipment requires less fuel.
Read more: Downstream Digitalization: Maximize throughput by ensuring operational excellence
Here are some examples of how Cognite’s solutions can improve your maintenance activities:
Scalable pump performance overview. In one specific use case, Cognite created a pump performance dashboard with all the relevant data that a company’s first-line support engineers require to do their jobs, including pump performance (both real-time and historical), events related to the pump (e.g. work orders), and estimated time until oil changes based on runtime. This solution enabled the company to extend the lifespan of its centrifugal pumps by 20%, improving planning and reducing waste by taking a more proactive approach to maintenance. With Cognite Data Fusion®, the company was also able to scale these dashboards across multiple pumps with minimal effort and make the data readily available for internal or external use.
Hybrid ML-powered reboiler monitoring. Cognite products help monitor reboiler health by identifying operational conditions that influence the heat transfer coefficient degradation rate of reboilers and predicting when maintenance is recommended or required. Using hybrid ML -- a combination of data science and physics -- to predict when to clean the reboiler, and machine learning to estimate reboiler heat transfer coefficient degradation over time, Cognite greatly simplifies the integration of data from physics simulators to enrich the analysis in confluence with live and historical data from different sources. In one example where this solution was implemented, engineers found they had enough lead time to plan maintenance activities in advance, helping the company avoid downtime. The solution can also be applied to many other types of equipment to give a holistic picture of when the equipment might need maintenance. This can increase production, optimize refinery operations, and boost uptime.
Oil separation quality monitoring. In another instance, Cognite, using the contextualized data in Cognite Data Fusion®, set up an automated root cause analysis system that improves oil separation quality monitoring. The system, built by Cognite's and a customer’s subject-matter experts, uses a live hybrid ML model to identify bad actors of poor oil separation and provide recommendations for how to improve the separation process. By comparing the suggested bad actor with relevant data, end users can validate whether a component is a true bad actor and perform mitigating actions accordingly. This enables engineers to better plan production, as they can change settings to reduce the chance of quality-related problems before they arise. The oil-water separator analysis system helped boost the uptime of the plant, and increased the equipment’s efficiency and throughput, saving the customer an estimated $6 million a year. This is a scalable solution that can be deployed using Cognite Data Fusion® to gas-oil separation plants (GOSPs) struggling with crude oil quality.
Maintenance prioritization. To streamline maintenance and inspection activities for an operator, Cognite built an application that aggregates alarms across all machines and applies custom rules so that the operator’s maintenance team could easily identify hot spots and prioritize actions based on severity, duration, interruption, and more in a 3D interface. With better marking and collection of data, the application further refines decision-making, providing more reliable estimates on the time needed to fix issues and which machines have reoccurring issues, which helps maintenance teams optimize maintenance campaigns. Visual contextualization of SAP maintenance data drastically reduces time spent planning maintenance work orders, increases efficiency, and democratizes access to data that experts can use to increase equipment performance.
These are just some examples of how improved access to data can enable smarter maintenance work. By making industrial data more accessible and meaningful to humans and machines, Cognite helps downstream operators draw insights from their data and unlock opportunities in real time.
Curious if Cognite Data Fusion® can help your organization? Book a free demo here.
This post is an excerpt from the white paper Downstream Digitalization.