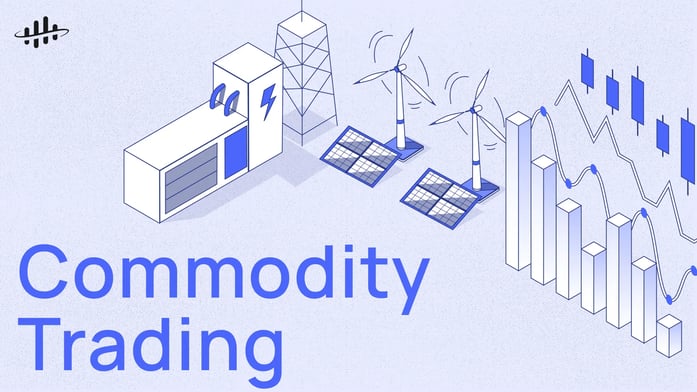
Commodity markets are in constant flux, changing in response to short-term supply chain crises and the longer-term global energy transition. Specialized operators—including commodity producers, large consumers, and traders—are looking to commercial optimization, also known as trading, to capture commercial opportunities, manage risk, and strengthen their competitive position in the market.
However, with increasing market complexity, the race to stay ahead of the competition is increasingly becoming a game of data. The market leaders of tomorrow will be those who can make the best data-driven decisions and manage the growth in data in the most efficient and flexible way.
Read also: How Utilities Can Manage Unprecedented Operational Change With DataOps
When thinking about advanced data management in trading, you may think of high frequency trading or "quants" such as those in Michael Lewis's book Flash Boys. In reality, most data management happens on much longer timescales than fractions of a second, and decisions are made to optimize operations of large asset portfolios longer term rather than primarily short-term financial arbitrage.
Market fluctuations in the commodity markets impact all of us, as seen from recent and current market upheavals. It’s not just the most active market participants who need to manage market data—it’s anybody with significant exposure to energy prices.
Access to high-quality data, and being able to use that data to create actionable insights, is critical to all managers of energy exposure along the value chain. Any consumer or producer of energy needs to understand their own exposure and potential gain from adapting to market changes.
At Cognite, we’re seeing five trends that highlight the data challenges that consumers, producers, arbitrageurs, and others often face in the commodity markets:
![]() |
1. Rapid increases in the number and availability of new data sources are accelerating the complexities of managing data and analytics in global markets |
With market dynamics such as the ongoing energy transition and consequent sector coupling (for example transport, industry, and other sectors increasingly interlinked through electrification), there are few signs to suggest that the acceleration of complexity will slow down any time soon.
This growing complexity puts significant pressure on commodity producers, traders, analysts, and IT organizations alike. Changing market dynamics also creates internal complexity for any company involved in marketing or trading of any commodity, with new data and alternative sources always on the radar of a commercial organization in search of that slight competitive edge.
![]() |
2. Reliance on legacy systems means participants in high-paced commodity markets struggle to make data relevant and actionable |
More data sources mean more data. Many companies are wrestling with mountains of data they already collect while trying to integrate new data into existing models and tools. However, with millions sunk into legacy systems that hold years of data, and without contextualizing and transforming data into a standardized model, updating new systems becomes a Sisyphean task.
In commodity markets, market analysts and quants have responded by trying to double down on artificial intelligence and black-box algorithms. Yet with data sources still fragmented, the engineering task of structuring data for processing pre-algorithm becomes a significant bottleneck.
![]() |
3. Participants in commodity markets need to accelerate their data management and digital development just to keep up with technological improvements |
With effective data operations and contextualization, many market operators are now experiencing how proprietary solutions built on top of out-of-the-box data engineering (rather than black-box data science) can give them a competitive edge.
Digitalization and integration of data sources accelerate the opportunities for companies to capture value. Commodity markets have a long history of being on the forefront of using information as a competitive advantage, but in an environment where more and more data becomes available, operators have to continuously innovate just to keep moving.
Not long ago, a commodity trader would primarily try to track the flow of products and commodities using an elaborate network of agents, trade documents, and human sources. Though human networks remain important, a large share of today’s commodity trade flows are analyzed using a vast landscape of digital resources, from real-time satellite data, consumer sentiment analysis, advanced sensor data, continuous traffic monitoring, and other alternative data sources.
The competitive edge from having the latest analytics or out-of-the-box solution is becoming increasingly time-sensitive. New models must be adapted and updated as soon as they are built. To stay ahead, developers need a data architecture that enables rapid in-house iteration by analysts and data scientists.
![]() |
4. Existing data solutions in commodity markets are often designed as one-stop single solutions or platforms, with little room to create a proprietary competitive edge |
Without the required data infrastructure and architecture in place, many participants in the commodity markets have in recent years looked for a silver bullet—the single all-encompassing platform offering—in an attempt to keep up with the rapidly evolving market. These solutions promise ease of use, competitive functionalities, and frequent updates, in exchange for a "black hole" solution in the back-end that pulls in data and analytics with limited room for escape or ability to be used in alternative ways.
However, few solutions offer access to the underlying calculations or data sources, and provide little in terms of flexibility and customization. Users wait around for regular updates, but these updates are not always relevant for individual users or quickly become outdated compared to competitive solutions. Knowing that the business model of solution providers typically revolves around scale, the solution a user purchases from a vendor is typically also sold to most direct competitors, meaning that the solution provides more of a license to play than an edge in a competitive market.
![]() |
5. Continued growth in market complexities will require that IT platforms and data architectures are designed to remain flexible and agile |
Flexibility and agility all have their foundations in a modularized (rather than a monolithic) approach to designing a data architecture and analytical landscape. Rather than looking for the one platform that will solve all their problems, trading companies are increasingly looking to purchase specific technical functionalities and capabilities from vendors. Being able to process raw data into contextualized insights in an efficient way enables traders to surf on top of the data tsunami, and helps analysts, quants, and IT organizations to rapidly develop new analytics when the market faces a new disruptor.
At Cognite, we work with commodity companies, large industrials, and traders alike, providing the data foundation required to contextualize new and existing data, integrating in-house analytics with third-party vendor offerings, and developing proprietary solutions with our Industrial DataOps platform, Cognite Data Fusion.
Industrial DataOps is the technical field of providing architecture for data and reducing the time required to build and iterate on new and more advanced solutions. This emerging discipline is quickly becoming one of the single most important vehicles for turning industrial data into tangible value and powering asset-heavy industries’ digital transformations.
Together with companies actively involved in commodity markets and other industries, we can provide the data operations and functionalities that enable users to quickly integrate new data sources, contextualize the information, and immediately convert data into actionable insights. By providing the data infrastructure and engineering capabilities, Industrial DataOps helps companies develop their own models in a highly flexible and adaptive way, enabling them not just to keep up but to get ahead of competitors struggling with legacy systems, black-box solutions, or heavy DIY processes.