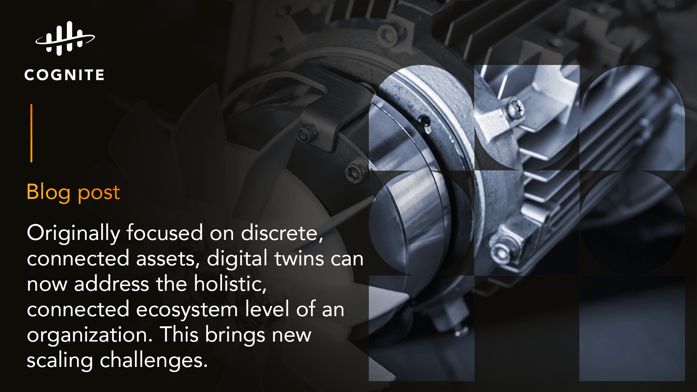
For some time, the notion of digital twins has been ubiquitous in exemplifying the potential of digital technology for heavy-asset industries. With a digital representation of a real-world system of assets or processes, we can apply simulation and optimization techniques to deliver prescriptive decision support to end-users.
Simulation Digital Twins help industries to make decisions in an increasingly complex & uncertain environment, to balance competing constraints (revenue, cost, efficiency, resiliency, carbon footprint, ++), and to react quickly and adapt with agility to real-world changes.
In this article we are describing solutions that combine the capabilities of Microsoft Azure Digital Twins, Cognite Data Fusion and Cosmotech Simulation Digital Twins. In an integrated solution, Azure Digital Twins provides a digital twin model that reflects real time state from sensors and other real time source and orchestrates event processing. Cognite Data Fusion (CDF) delivers integration of schemas and metadata from IT, OT and ET data sources, including the generation of models and twin graphs for Azure Digital Twins. The Cosmotech Simulation Digital Twin platform adds deep simulation capabilities in a scalable, open framework.
The challenge of scale
Originally focused on discrete, connected assets, digital twins can now address the holistic, connected ecosystem level of an organization. This offers significant value creation possibilities, but also brings new scaling challenges. While monitoring millions of individual assets and predicting potential failures is a standard capability of IoT platforms, the next level challenge is how to offer the capability for users to rapidly predict the impact of unexpected events on their business, so they may make robust and optimized decisions that account for competing constraints. Delivering such capabilities requires deploying digital twin simulation at the ecosystem scale, and this is directly related to bridging data operations capabilities with simulation. Typically, we see three such challenges:
- Heterogeneity of data sources
- Evolving ontologies
- Multi-scale use-cases that operate on multi-granularity twins
Figure 1: DataOps challenges in the Asset Management context
Heterogeneity of data sources
The necessary input data typically spans a variety of source systems and data types. For example, a maintenance optimization use case would rely on
- physical asset data such as asset register descriptions and sensor information, but also the outcome of calculations such as a health index.
- enterprise asset data, such as maintenance policies, inspection results, and maintenance events
- other contextual data, such as business targets, sales demand forecasts or production value.
Furthermore, these data sources can rely on various IT systems that could be different from one factory or site to another, bringing an even greater complexity of implementation and maintenance to keep the digital twin in sync over time and at an acceptable cost.
The heterogeneous source system landscape makes accessing relevant information challenging and is seen as a substantial bottleneck when scaling a given solution across a customer’s asset portfolio.
Evolving ontologies
Deploying digital twins in an organization is a journey. Digital twin ontologies that describe data evolve in accordance with the digitization roadmap of the organization. As an example, a Transmission System Operator (TSO) initially modeled overhead lines as monolithic assets in their asset registry but some years later the same concepts were described in terms of steel towers, fittings, steel lattice, foundations, and cables that are described by the number of their ruptured strands. Another example for this same TSO starts with a simplified twin of its operations, considering at first simplified outage management, based on individual asset criticality classification. The twin then evolves over time to a more precise network model inside the twin, that offers better outage management, and maximizes energy transported while controlling risk.
Multi-scale use cases that operate on multi-granularity twins
Various digital twin initiatives necessarily operate on data at different levels of aggregation. The lack of an integrated value proposition, relying on ad-hoc dataOps is error-prone and induces a high cost to build and maintain. There is typically no solution for users to launch scenarios at all scales, making sure data is coherent and maintained over time.
In the manufacturing industry, offering a “network” of Digital Twins simultaneously requires:
- a fine-grain value proposition for operations inside the factory (usually OPEX + side adjustments on CAPEX)
- a coarse grain value proposition for the central management (sustainability, business growth strategy, balancing CAPEX with OPEX as well as balancing growth with sustaining CAPEX)
In the utility space, a TSO needs to balance regional planning needs with central asset management requirements. Both simulation twin solutions have their scope of decisions, relying on different data granularity, but both require the same ontology to make sure strategy and operations are aligned.
Industrial DataOps
Cognite Data Fusion offers an industrial data operations platform that serves contextualized data that originates in a wide range of siloed source systems and supports all relevant industrial data types - across OT/IT/ET systems. With contextualized data we mean that information across source systems and data types is semantically connected and queryable by humans and machines. For example, a wind turbine is broken down into its sub-components, enhanced with maintenance history (ERP data), sensor information (SCADA data), 3D models and images obtained from inspection routines, but also linked to any technical documentation, engineering diagrams, and more.
Learn more: Contextualized data and digital twins amplify digitization value
At the core of Cognite’s offering is an industrial knowledge graph that establishes relationships with informational entities across source systems and data types. This provides a dynamic, easily governable semantic data layer that makes data meaningful for humans and machines and is exposed through open APIs and SDKs to solutions that leverage this data to solve business problems.
Figure 2: How it all fits together to provide industrial digital twin applications
In practice, every solution has specific expectations and requirements to how data is supposed to be structured and modeled, and to this end, Cognite Data Fusion provides a flexible data modeling framework that allows for such different perspectives into data to be clearly described.
Figure 3: Data models provide different perspectives across sources, domains, and solutions.
While the source data model liberates data from a variety of source systems it also maintains query ability in Cognite Data Fusion through the same API interfaces. The domain data model synthesizes information across data sources and allows for a detailed description of an asset or process that can be continuously enriched as new information and data sources become available.
A solution-specific data model imposes a schema that the application can validate and rely on and the instances of which are populated with the help of Cognite’s contextualization services in an automated way, leveraging a domain-guided machine-learning approach. Compared to the domain data model, which allows for a higher level of entropy and the representation of evolving ontologies, the solution data model is much more rigid while at the same time allowing for true scalability across two dimensions.
-
Scalability of one Solution is ensured through automated population of solution data model instances, e.g. scaling a maintenance optimization solution across the entire asset portfolio.
-
Scalability across a portfolio of solutions is enabled by immediate access to a wide range of data sources and the fact that application requirements to data are decoupled from the representation in the domain data model. This allows for use cases to be solved that require different levels of data granularity, e.g. plant-level maintenance optimization vs enterprise-level strategic planning.
Figure 4: Industrial DataOps scales solutions across instances and use cases.
Bottomline
To really deliver on the value potential of digital twins in an industrial context, prescriptive decision support is a key outcome that is enabled by Cosmo Tech’s simulation and optimization based solutions. There are, however, fundamental challenges when scaling Simulation Digital Twins across portfolios of instances and use cases and addressing those challenges requires the strong Industrial DataOps foundation that Cognite Data Fusion provides.
Article originally published at: https://techcommunity.microsoft.com/t5/internet-of-things-blog/industrial-dataops-capabilities-to-truly-scale-simulation/ba-p/3440096