The value of data-driven decision-making in industry has gone mainstream. From OEE, Overall Asset Performance, quality optimization, energy efficiency, and equipment health to sustainability improvements, the value of enabling the wider organization to make data-driven decisions has been proven in countless ways.
To truly enable an organization this way, the old-fashioned data lake isn’t enough. The data needs to be put in context and be made meaningful.
Learn more:Contextualized data and digital twins amplify digitization value
That’s what digital twins do today. They serve data in a way that matches operational decisions that are to be made. As a result, each company needs multiple digital twins, as the type and nature of decisions are different. There’s a digital twin for supply chain, one for different operating conditions, one that reflects maintenance, one that’s for visualization, one for simulation—and so on.
What this shows is that a digital twin isn’t a monolith, but an ecosystem. To support that ecosystem, industrial companies need an efficient way of populating all the different kinds of digital twins with data in a scalable way.
Industrial DataOps, a discipline for industrial data management, can provide this data backbone for digital twin creation and management. With an efficient way to create digital twins, companies can enable more people across the enterprise to make data-driven decisions, putting the power of digital innovation into the hands of experts at plant floor operations, remote, across plants, in aftermarket services, and in R&D.
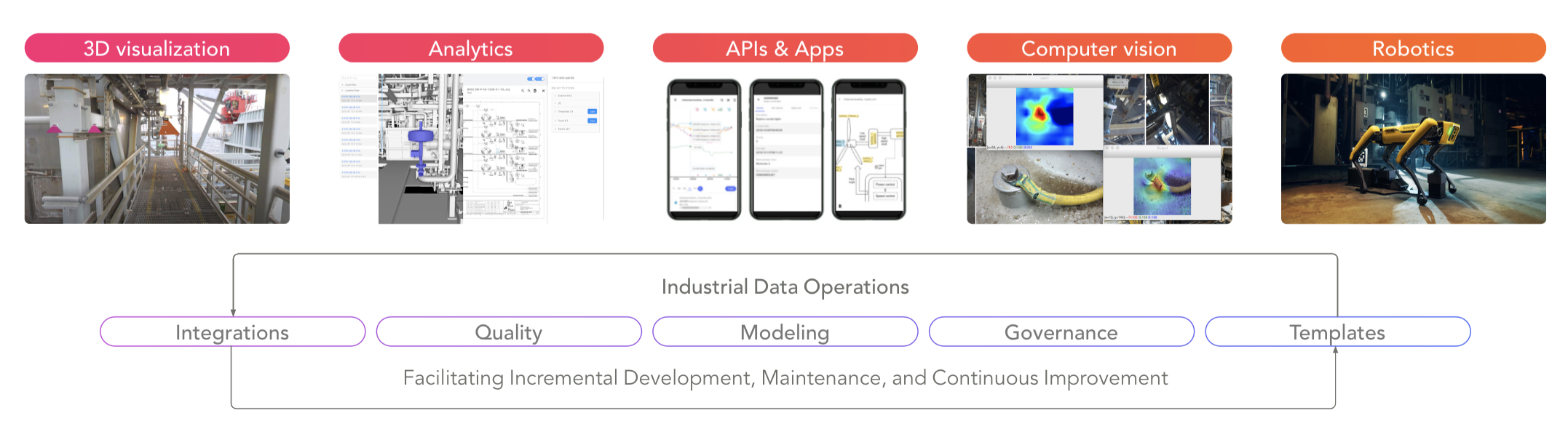
Figure 1: Equipping the process & capabilities that bring digital twins to life
From digital twins to ‘digital siblings’
The composable way of thinking about the ecosystem of digital twins supports how businesses in general need to be run across engineering, operations, and product. In the words of Rick Franzosa:
“Stuart Kauffman in 2002 introduced the ‘adjacent possible’ theory. This theory proposes that biological systems are able to morph into more complex systems by making incremental, relatively less energy-consuming changes in their makeup. This is a construct that applies to how smart manufacturing benefits are achieved. The adjacent possible means making an incremental improvement that then uncovers another avenue for improvement.
Manufacturing is a discipline that tends toward being risk averse, rigid, disciplined. But concepts like continuous improvement and bimodal, as well as the never-ending drive for higher quality, lower cost and personalized rapid delivery are inching manufacturing toward a more composable mindset to meet these challenges.”
What is needed is not a single digital twin that perfectly encapsulates all aspects of the physical reality it mirrors, but rather an evolving set of “digital siblings.” Each sibling shares a lot of the same DNA (data, tools, and practices) but is built for a specific purpose, can evolve on its own, and provides value in isolation.
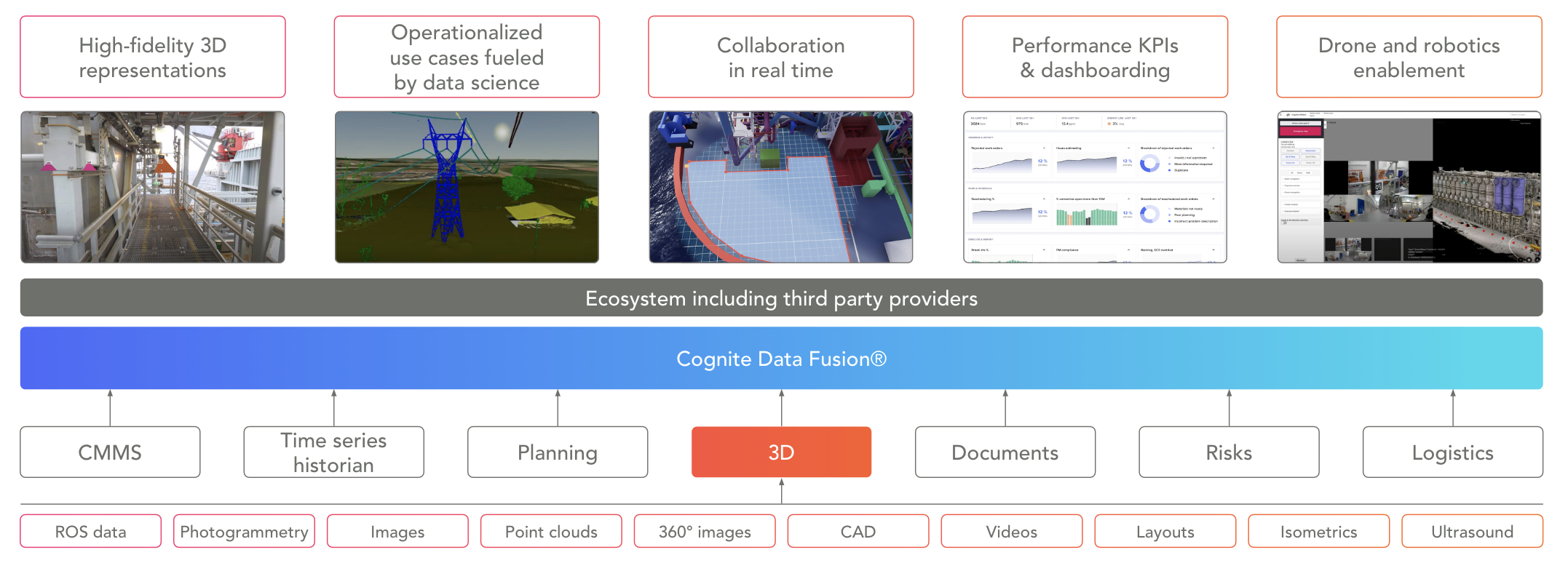
Figure 2: Suite of specialized digital twin tools for ongoing enrichment
The family of siblings accurately reflects the various aspects of the physical reality that provide value, and provide more autonomy and local governance than the building and maintenance of a single canonical digital twin.
Solving the data problem with a data backbone
This composable way of thinking about digital twins puts the spotlight on what's blocking enterprise business value today: How can we efficiently populate and manage the data needed for the digital twins across the inherent entropy and ever-changing nature of physical equipment and production lines?
The answer lies in how to think about the data backbone supporting the digital twins. The data backbone needs to be run in a distributed but governed manner known as Industrial DataOps. This data management discipline focuses on breaking down silos and optimizing the broad availability and usability of industrial data generated in asset-heavy industries such as manufacturing.
Learn more: What is DataOps? 5 things industrial leaders need to know
At the core of how to efficiently accelerate the initial rollout and the incremental improvements needed for digital twins is expert engagement and enablement. It’s necessary to engage and build on the competency of the operators and subject-matter experts who know their equipment and production lines the best, and to drive efficient data-grounded collaboration for these roles.
This data problem is too big for an IT department alone to solve, and a data lake isn’t enough to involve operators on their own terms.
Read also: Think beyond data lakes: data products and data value measurement
The data backbone to power digital twins needs to be governed in efficient ways to avoid the master data management challenges of the past—including tracking data lineage, managing access rights, and monitoring data quality, to mention a few examples. The governance structure has to focus on creating data products that may be used, reused, and collaborated on in efficient and cross-disciplinary ways. The data products have to be easily composable and be constructed like humans think about data ; As a graph where physical equipment are interconnected both physically and logically. And through this representation select parts of the graph may be used to populate the different digital twins in a consistent and coherent way.
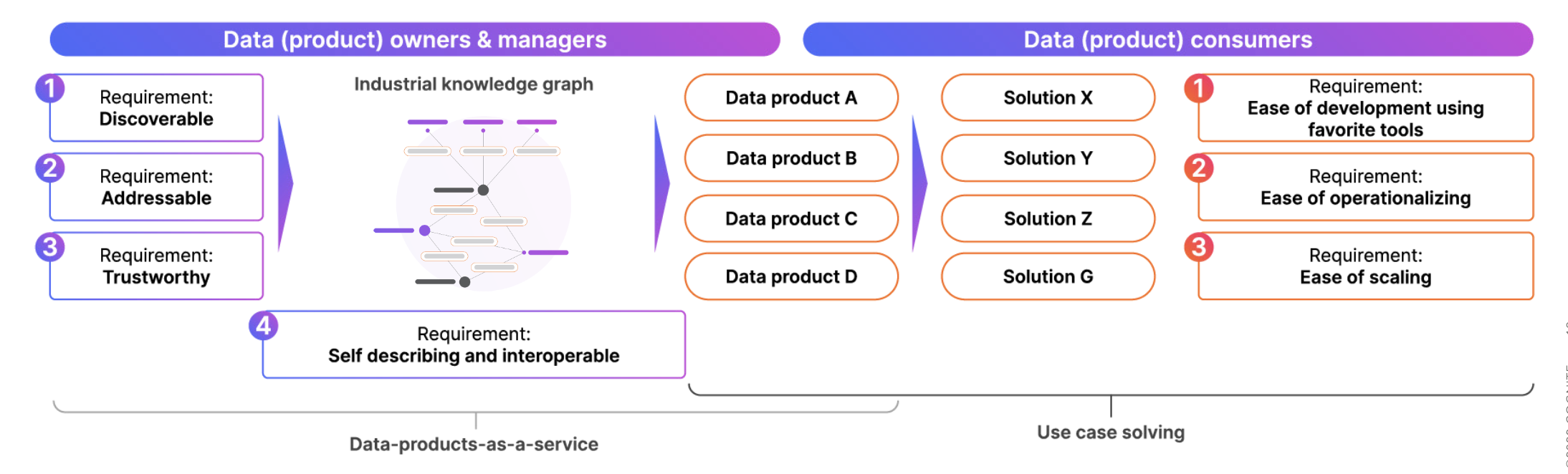
Figure 3: The shift from data availability to data products as a service is what will transform our data swamps into operational data lakes of real business value
One way of looking at governance is by separating the layers of governance structures and associated data management:
- Digital twin solution-specific data onboarding and governance, where operators and plant IT workers create data products that power operational decisions.
- Discipline-specific data onboarding and governance (for example operations, engineering, or product), where subject-matter experts and domain specialists create shareable data products.
- Enterprise-wide data onboarding and governance, where specialized data management and IT teams create enterprise-wide data products.
For each of these data products there’s a custodian shepherding the process. An enterprise-wide network of custodians is important to achieve the nonlinear scaling effect across sites, production lines, and equipment.
Build your data backbone with Industrial DataOps
Digitalizing how businesses are run isn’t about what technology can do—it’s all about having a product that makes it easy for everyone in an organization to create data products and make data-driven decisions. Convenience is the key concept to embrace.
To get this distributed model to work, the product should:
Define and update what data should be added to data products and how it relates to business needs from digital twins and solutions.
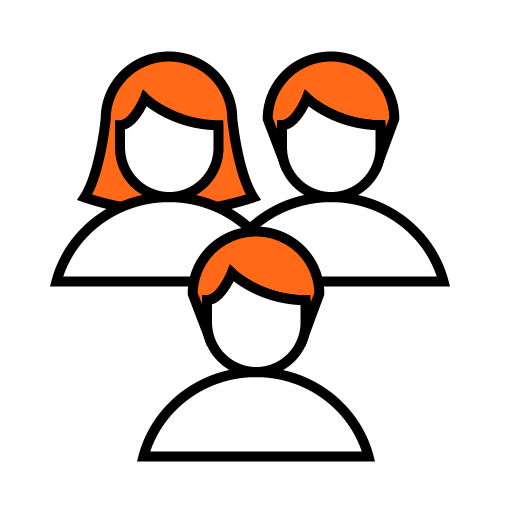
Define and monitor who should be able to access the data and who could be interested in it.
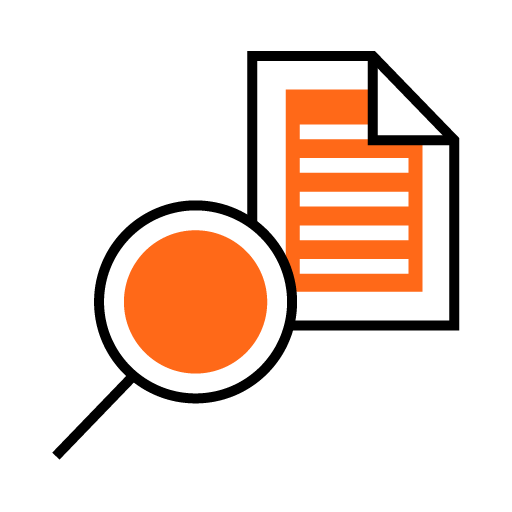
Define and monitor what data quality can be expected from the data in the data product
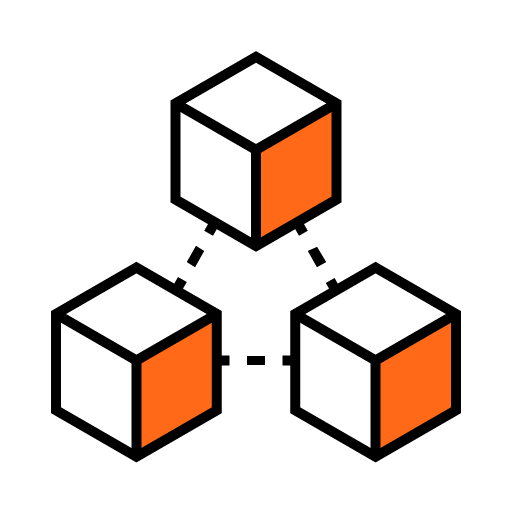
Define how the data product is related to other data products
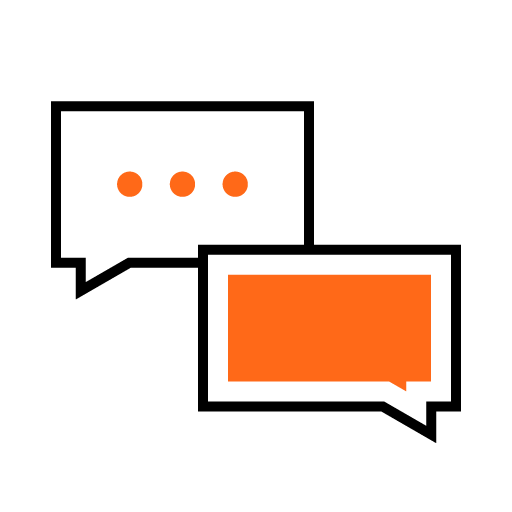
Keep communicating with the data custodian network to make sure that innovation and collaboration happens in efficient ways across the different data domains
Cognite Data Fusion, the leading Industrial DataOps platform, serves as the data backbone for digital twins for dozens of industrial companies around the world. The SaaS product serves data to a broad set of digital twin types in a way that secures rapid rollout to hundreds of sites, makes them efficient to deploy and operate, and secures support for lean processes when they’re in production.
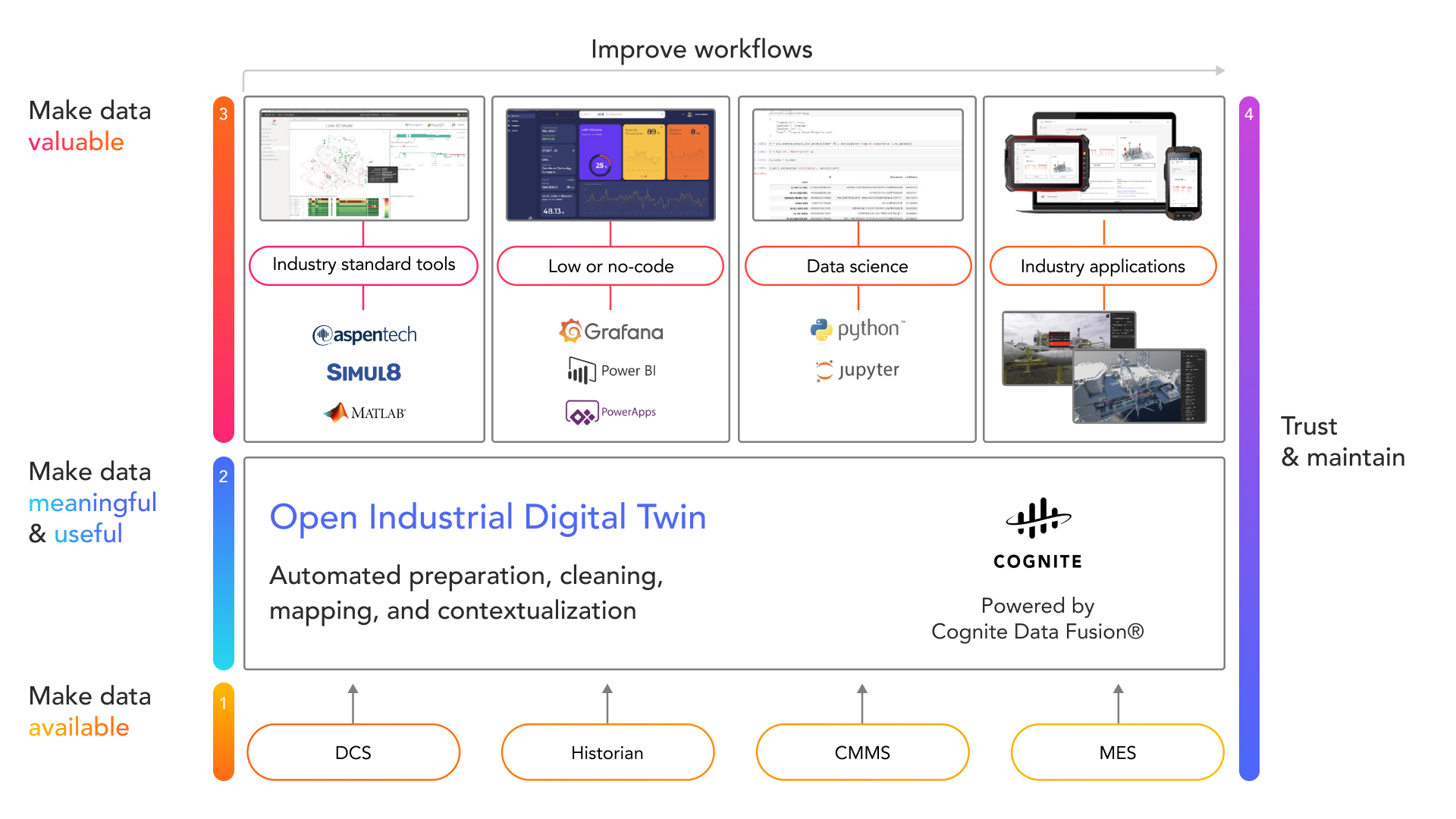
Concepts like data lakes, data fabrics, and data catalogs haven’t lived up to expectations when it comes to bringing together and serving the needs that depend on data coming from core engineering, operation, and product processes. Cognite Data Fusion serves this purpose. As the industrial digital twin depends on this kind of data, Cognite Data Fusion secures business agility and empowerment as digital twins are deployed across sites and business units.
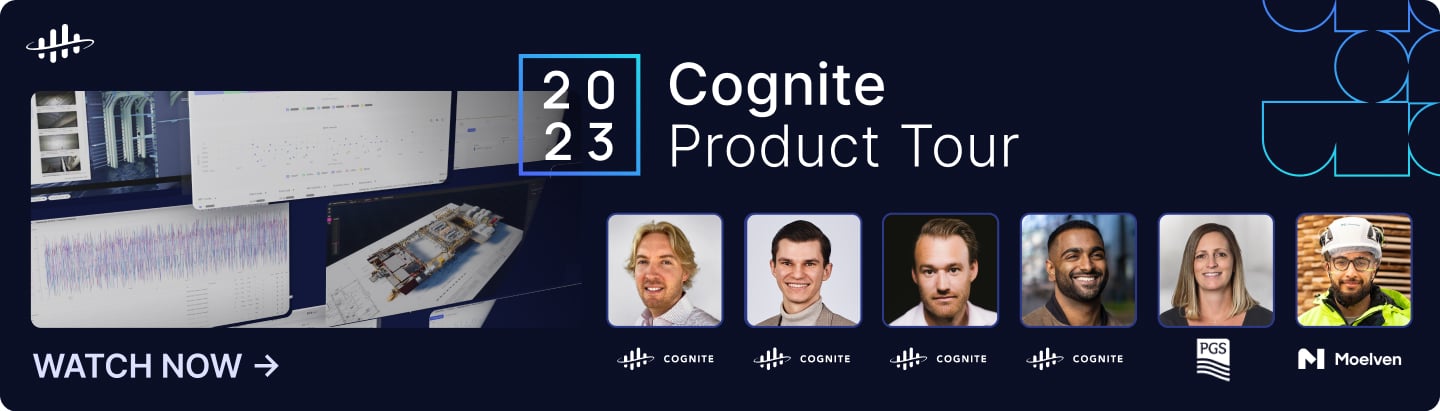
About Cognite
Cognite is a global industrial AI Software-as-a-Service (SaaS) company supporting the full-scale digital transformation of heavy-asset industries around the world. Their key product, Cognite Data Fusion® (CDF), empowers companies with contextualized OT/IT data to drive industrial applications that increase safety, sustainability, and efficiency, and drive revenue.