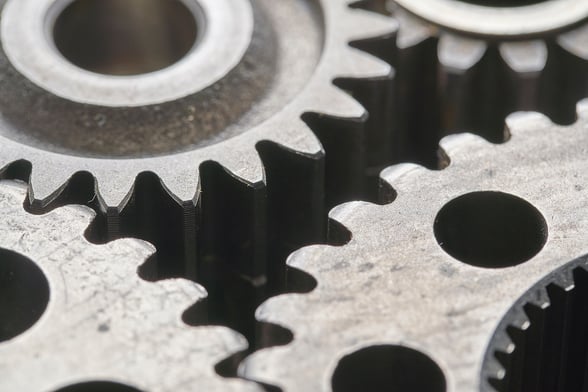
How a combination of new tools, effective data flows and stress testing will help industries operationalize their best ideas — fast. Reflections from Cognite Co-Founder and CTO Geir Engdahl.
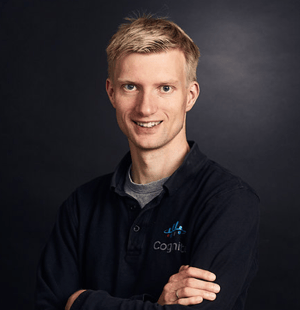
Since the inception of Cognite, I have learned a great many things about industry and digitalization. Coming from a consumer internet background, I naively thought collecting data would be a walk in the park. It was not. So we spearheaded a drive to free data in order to make it accessible and to make collecting it more effective. We called this the “Data Liberation Front”. It resonated within industry and soon grew outside Cognite, too.
After we found a way to liberate data, I, again, thought that using this liberated data would be a walk in the park. It was not. So we built contextualization tools to connect the data into an intuitive data model. Then, operationalization presented another set of challenges, which I’ll discuss more below. Surprisingly, or perhaps not, we found that the questions we get from industrial companies are very similar, indicating to us that productized solutions can be built, so industry can avoid the cost of everyone inventing their own cogwheel.
Particularly now that purse strings have been tightened, the stakes in turning a promising concept into an operational product or service are particularly high. Too often, we have seen great proof of concepts failing to operationalize. A dashboard showing the output of a data science model is a good example. In its first iteration, it might be based on static data with a computation run once on a data scientist’s laptop. Operationalization is about going from that to something that is live and advising an operator on how to best run a piece of equipment, for instance. Any digital project which has not grown into that, is a cost center and doomed to die.
The great challenge industry faces is speeding up the time it takes to turn a great proof of concept into a quality-controlled, real, cost- and time-saving product (or service or process). To tackle this, we need to do three things:
1: Build a safehouse for models
The data science model is a piece of computer code that needs to be run regularly, automatically and on evergreen input data. Taking the computation environment first, the data scientist’s laptop is not a great place to run the model once it is in production. It is too brittle and provides low availability, not to mention the security concerns. A model-hosting environment, like Cognite functions, which is in beta for select customers, where code executions can be scheduled, logged and monitored, is needed. There are many more alternatives, like Azure functions, Google cloud functions and AWS lambdas, targeted at a wider audience than heavy industry.
2: Ensure data flow: avoid ‘garbage in, garbage out’
A model is only as good as its input. So the next thing we need to do is ensure that fresh, accurate and complete data keeps flowing to the model. Industrial data is complex, often originating from several dozen different source systems, transformed into common information models and formats and then made available to data consumers. To ensure the integrity of the data, companies must have proper governance on who or what can write to each data set. In Cognite Data Fusion®, we introduced Data Sets earlier this year, which does just this.
3: Stress test data collection processes and sensors
A digital representation of what is going on in your plant may be broken even if its lineage is intact, so it’s critical to detect and alert on the most common reasons why sensor data does not match reality. Causes could be problems with upstream data collection processes, sensor flatlining or dead sensors. Companies should continue to expand the types of problems they detect and in supporting custom code to delve deeper into more specific problems.
We are bringing these and several other functionalities together - and fast - because we are committed to helping industry operationalize great ideas better and speedier than ever before. These days, we have zero time to waste, so let’s get to work.